The European Policy context: strategies and legislation
Artificial intelligence (AI) and big data represent a highly relevant and priority topic for policy, research, and industry globally. More precisely, the quality of data used for building and training AI-based algorithms is subject to high attention as it can have very serious consequences that range from missed business opportunities or lost in revenues for companies to much more problematic damages when ethical issues arise.
Data quality can refer to a broad range of data problems, such as representativeness, quantity, inconsistency, ambiguity, inaccuracy of data. Regardless of the type of problem affecting data, it is widely acknowledged that the quality of an algorithm and its outcomes or applications strictly depends on the quality of inputted data. If low quality data is used, therefore, questionable or poor decisions could be made. For example, privacy issues could arise as a result of low quality data, or biases in data could lead to discriminatory consequences. Let us think, by means of mere example, to the case of voice recognition systems trained on specific types of voice or accents that could not recognise different voices and perform poorly when used in different contexts [1].
For these reasons, the topic of data quality is mentioned repeatedly in policy documents in relation to the use of AI. Indeed, the EU’s approach to AI precisely centers on excellence and trust, aiming to boost research and industrial capacity while ensuring safety and fundamental rights which can be guaranteed by investing in the implementation of appropriate data governance and management practices to provide high quality training, validation and testing data sets.
The European AI Strategy aims at making the EU a world-class hub for AI and ensuring that AI is human-centric and trustworthy also via the European Commission’s High Level Expert Group on AI set up in 2018, which has published ethics guidelines on AI and provided a set of measures to build trustworthy AI systems, i.e. able to ensure protection of human rights as they perform properly [2]. These aspects are all crucial to data quality management because, as said, AI algorithms’ performance does depend on the quality of data. A full package of measures was released in 2021 by the European Commission to drive towards AI excellence through concrete rules and actions including, among others, the AI Act.
The role of standardisation
Among the measures that can help improve data quality in AI, standards play a major role. Standards and codes of conduct can help AI systems operate in a safe and reliable manner thus preventing any unintended adverse impact.
A lot of standards exist, for example, for design, manufacturing and business practices, as well as for quality management system for AI users, consumers, organisations, research institutions and governments that offer them the ability to recognise and encourage ethical conduct through their purchasing decisions. Current examples are e.g. ISO Standards (ISO/IEC TR 24028:2020 Information technology — Artificial intelligence — Overview of trustworthiness in artificial intelligence) or the IEEE P7000 standards series on Ethics in Action that touch also transparency policies and data quality aspects, all relevant also for the set-up of AI algorithms.
Several Technical Committees and Working Groups from European and International SDOs are active in the field of AI, Data quality and Ethics. A non-exhaustive list of such expert groups include:
- CEN-CENELEC JTC 21 ‘Artificial Intelligence’
- ISO/IEC JTC 1/SC 42
- ETSI Industry Specification Group on Securing Artificial Intelligence (ISG SAI)
More information on the existing standards in the AI domain can be found in the Landscape Analysis report produced by the Technical Working Group on AI set-up by the StandICT.eu project and available at this link.
[1] Read more here: Data quality and artificial intelligence – mitigating bias and error to protect fundamental rights
[2] Read more here: Ethics Guidelines for Trustworthy AI
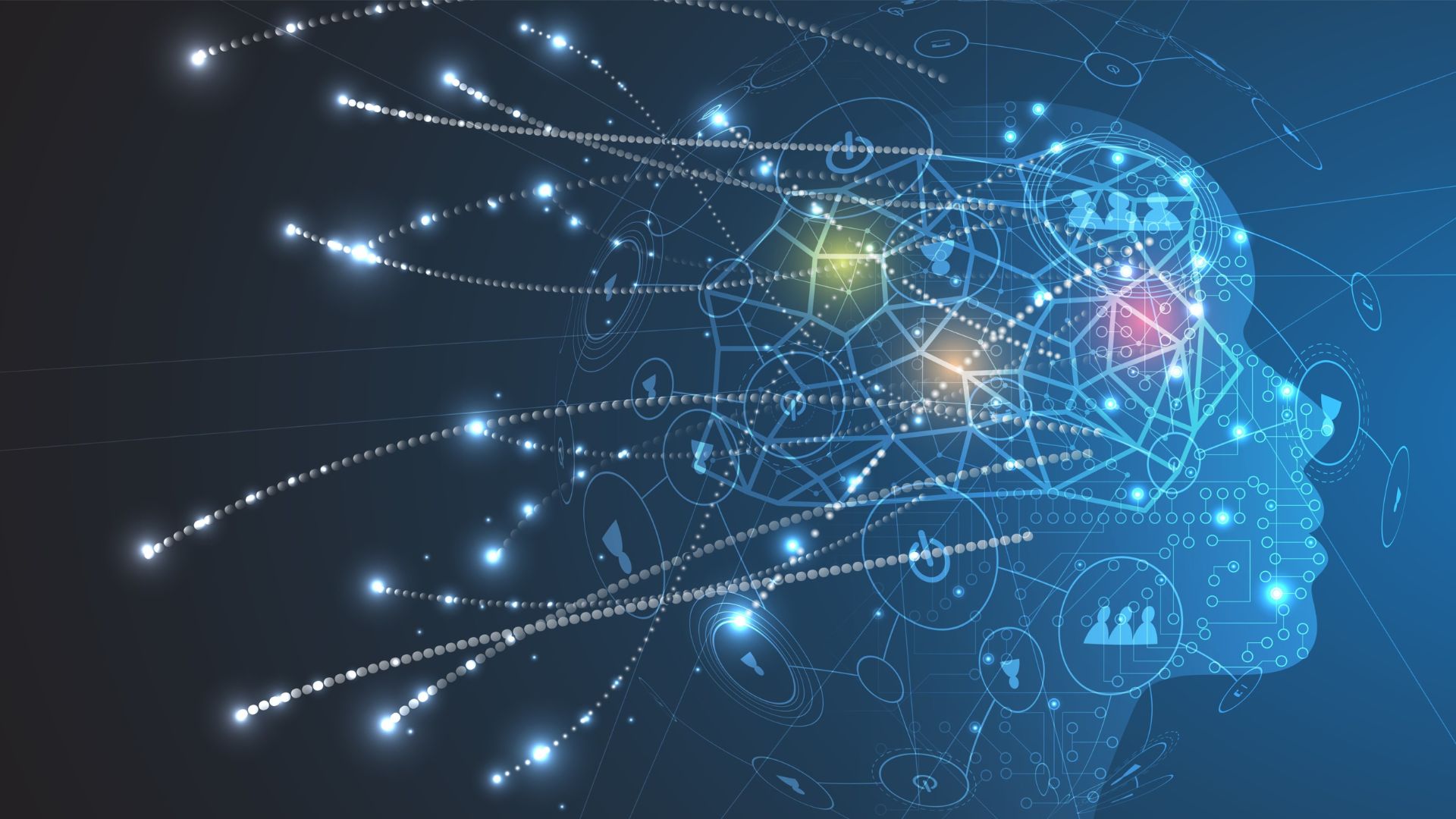